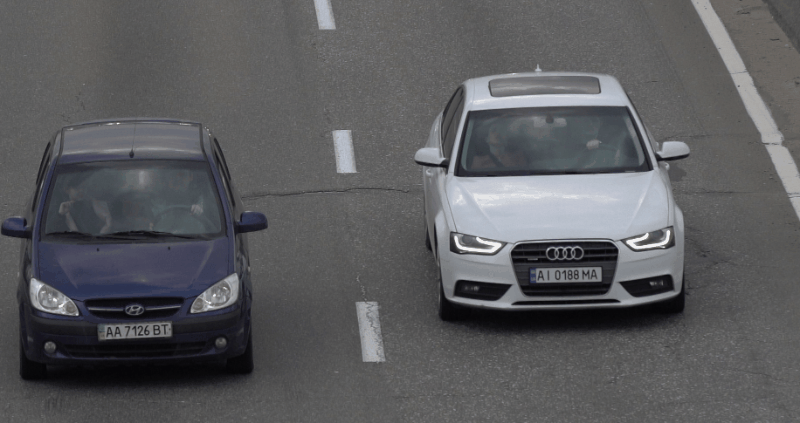
To be a pioneer in this evolutionary business niche and to hear the customer's needs of the traffic segment are the FF Group mission.
Using great experience in license plate recognition we have expanded the features of cars' searching on the EDGE without using a neural network.
We took the Axis camera with a strong ARPEC 6 chipset and implemented the first application with the automatic vehicle license plate recognition and classifying type, make (brand), model, and detect the vehicle color onboard of the camera.
New technology got the name VMMCR (Vehicle make, model, and color recognition). Let's see how we create this magic on the EDGE.
Business requirements
Firstly, our R&D team has formulated the following product requirements:
1. Desired car features
Type (car, truck, bus ...)
Brand - (Honda, Volvo ...)
Color (red, blue ...)
Car model
These features were sorted according to priority based on our customers’ survey.
2. Accuracy
The technology must be accurate enough for solving the following customer's tasks such as searching for stolen cars by color and make (when a license plate could be changed to another);
or to classify cars in the bus lane, to separate buses from other vehicles.
Our internal goal was to reach 95% on the test dataset.
3. Platform and performance.
The majority of IP camera platforms do not support hardware accelerators for launching neural networks. So FF Group technology should work on ordinary ARM processors and be fast enough to classify cars in real-time in standard city conditions (two lanes - 60 km/h).
How it works and existing restrictions
For its own classify the vehicle has to be found in the video stream (so-called vehicle detection). Such a difficult task needed an effective technical and economic approach.
So, we decided not to develop a new algorithm but to use the current car license plate detector with an accuracy of more than 97%. In addition, new car features without the license plate detector not in demand in the traffic market.
In order for the algorithm to classify all vehicle parameters quickly and efficiently, including on EDGE, we used our own approach (know-how), and we made a small breakthrough.
The first step was to cut out the image around the detected license plate. Next, we identified in the original bitmap (picture) the key points of the car in our own mathematical way. Then, they were transformed into our own and new feature space, where FF Group algorithm quickly and easily classifies the car. So, work in such a space has significantly increased the speed and classification accuracy.
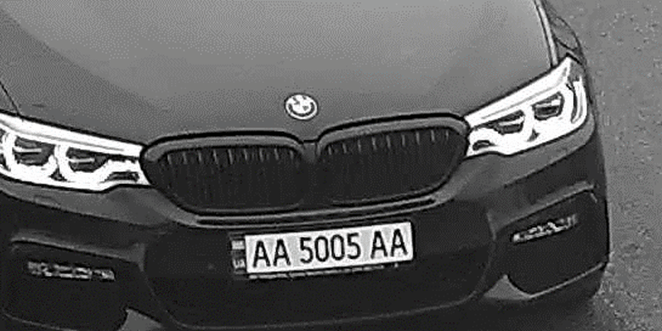
So what we have at the moment (March 2020, current release 1.4)
· 632 car classes;
· 74 brands;
· 6 basic vehicles’ types;
· 10 primary colors;
· VMMCR technology works only during the day (Modern IP cameras do not allow to see the contours of the car at night)
· front view only;
Why VMMCR doesn't he work at night?
The first issue. The license plate is clearly visible in the good IR illumination, but classify the car is not impossible.
The second issue. The license plate is clearly visible in the good IR illumination, but classify the car is not impossible.
The third issue. The license plate is clearly visible in the good IR illumination, but classify the car is not impossible.
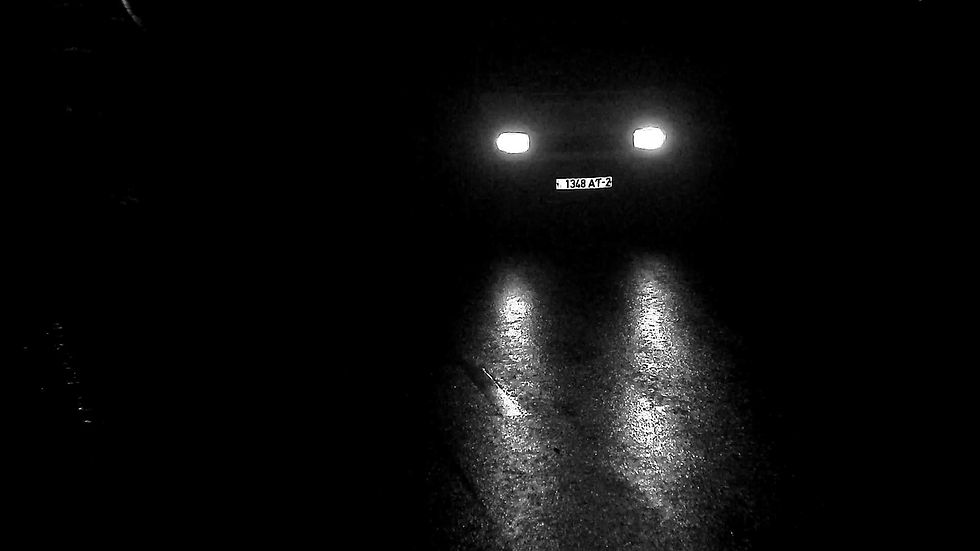
Field test and cases
VMMCR has constant proof of working in real conditions and not only on our data sets. It is the full-scale tests and customer reviews.
Let’s dwell on three examples from real installations in different countries and with different points of view. Accuracy in these locations is achieved from 92% to 95%:
1. Italy. One lane. Left view.
2. Czech Republic. Two lanes. Central view.
3. Ukraine. Three lanes. Central view.
Of course, such accuracy is not always achieved at the first try. Especially in regions where cars distribution by make and model differs from our current dataset and needs to be upgraded.
And short summary
Using rich FF Group R&D experience and own mathematical development the ANPR market gets outstanding and unique LPR and all vehicle parameters classification technology.
We achieved stated accuracy indicators in a short period thanks to our own developed "fast approach".
Today VMMCR technology was implemented into existing FF Group products on the EDGE (onboard of AXIS and Hanwha cameras) and on the server product NumberOK (the Windows).
Comments and compliments are welcome in the chat :)
If you have a project just drop your comments under the blog or on the e-mail sales@number-ok.com.
Comentarios